Understanding the Limitations of Clustering Benchmarks for Text Data
Clustering is a fundamental task in natural language processing, which involves grouping similar texts together based on their content. Evaluating the performance of clustering models is crucial to understand how well they work and identify areas for improvement. The Meta-Learning for Text Classification (MTEB) benchmark is widely used for evaluating clustering models, but it has some limitations that need to be considered when using it for text data.
Firstly, the datasets in MTEB are relatively small with a maximum split size of around 26k samples. This means that real-world applications may involve larger datasets with a high degree of semantic variability, requiring models to perform extremely fine-grained clustering. In contrast, our proposed German benchmark is less diverse in terms of dataset diversity.
Secondly, the ground truth for MTEB is based on topical categories derived from the data, such as the scientific discipline of a publication or newsgroup. However, this may not always be accurate, especially when dealing with complex texts that span multiple topics. Moreover, we only consider samples with one top-level and up to two second-level genres, which might limit the accuracy of the ground truth.
Lastly, MTEB uses a V-measure evaluation metric, which measures homogeneity (clusters contain only one class) and completeness (clusters contain all classes). While this metric is widely used in text classification, it may not be suitable for clustering tasks, especially when dealing with complex texts.
In conclusion, while MTEB is a useful benchmark for evaluating clustering models, its limitations need to be considered when working with text data. By understanding these limitations, researchers can better evaluate the performance of their clustering models and identify areas for improvement.
Computation and Language, Computer Science
Shaqiri swaps Inter Milan for Stoke City, becoming teammate of Arnautovic
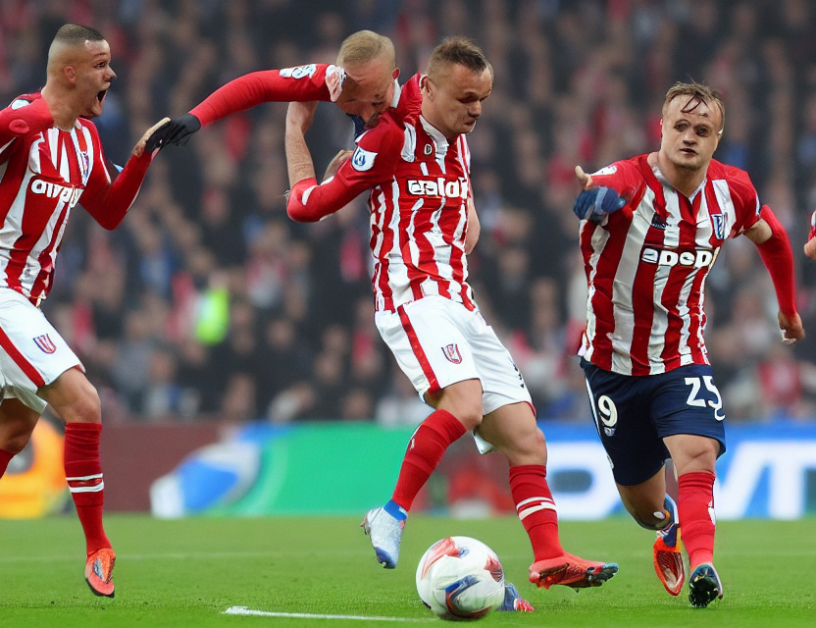