Survival analysis is a statistical technique used to study the duration of time until a particular event occurs. This approach is commonly applied in various fields, including medicine, engineering, economics, and sociology. The goal of survival analysis is to assess how different variables affect the length of time before an event happens. For example, a survival analysis could investigate the relationship between clinical factors like age, gender, or race and heart attack risk. In the medical field, survival analysis is often used to identify which factors are most strongly linked to disease recurrence.
The article "Big Data and Predictive Analytics: Recalibrating Expectations" discusses three streams of related work for survival loss functions in terms of technical components. These components include likelihood estimation, ranking, and calibration. The authors explain that these techniques are essential for accurately predicting the duration of time until an event occurs.
Likelihood estimation involves calculating the probability of an event occurring based on various factors. Ranking involves assigning scores to different variables based on their potential impact on survival time. Calibration ensures that the predictions made by the model are accurate and reliable.
The authors also provide examples of how these techniques can be applied in real-world scenarios, such as identifying which clinical factors most strongly influence heart attack risk or predicting the likelihood of disease recurrence based on various patient characteristics.
In summary, survival analysis is a valuable tool for understanding how different variables affect the duration of time until an event occurs. By using techniques like likelihood estimation, ranking, and calibration, researchers can accurately predict the timing of events and make informed decisions about disease prevention or treatment.
Computer Science, Machine Learning
Unlocking Survival Analysis: A Comprehensive Guide to Techniques and Applications
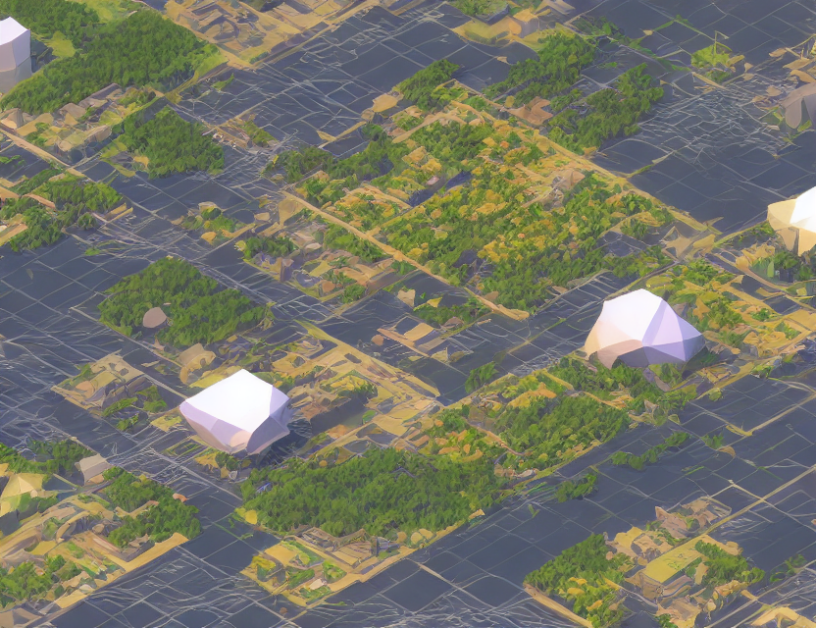