Sports analytics has come a long way in recent years with the availability of high-dimensional data. However, one major challenge that persists is the lack of understanding of complex statistical models used in sports analytics. These models are like recipes for cooking up insights into athlete performance and strategic dynamics in sports, but without the ability to understand how they work, it’s hard to make sense of the results.
The article tackles this challenge head-on by exploring the concept of interpretability in sports analytics. Interpretability refers to the ability to understand and explain the decisions made by complex models. The authors argue that while advanced algorithms like neural networks and ensemble methods have made tremendous progress in predicting athlete performance, they often lack transparency and are difficult to interpret.
The article highlights several challenges that hinder our understanding of these models, including feature redundancy and collinearity, the expansive nature of high-dimensional datasets, and the dynamic and context-dependent nature of sports. The authors also discuss the scarcity of research specifically focusing on the interpretability of high-dimensional sports statistics, particularly in competitive sports analysis.
To overcome these challenges, the authors propose a novel approach that combines feature selection and dimensionality reduction techniques to identify the most influential variables in complex models. They demonstrate the effectiveness of their approach using real-world datasets from basketball and soccer.
Throughout the article, the authors use engaging metaphors and analogies to demystify complex concepts. For instance, they compare feature redundancy and collinearity to duplicate recipes in cooking, where having too many identical ingredients can make it difficult to identify the most critical ones. They also liken high-dimensional datasets to a vast library with numerous books, making it challenging to find the one you need without a proper catalog.
In summary, the article provides a comprehensive overview of the interpretability challenge in sports analytics and proposes practical solutions to address these issues. By using everyday language and analogies, the authors make complex concepts more accessible and easier to understand, thereby contributing to the demystification of sports analytics.
Computer Science, Machine Learning
Unlocking the Secrets of High-Dimensional Sports Statistics: A Survey of Interpretability Techniques
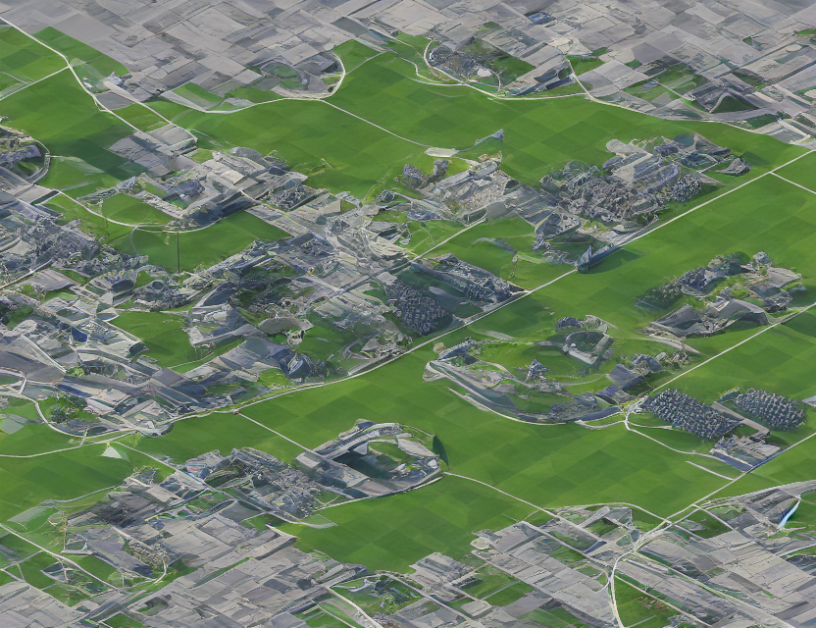