In this article, we explore the concept of explainable artificial intelligence (XAI) in the context of a multilayer case. XAI is the ability to provide clear and understandable explanations for the decisions made by an AI system. The authors propose a novel framework called GNN-based ATR (Automatic Target Recognition), which combines the strengths of graph neural networks (GNNs) with the explainability of XAI.
The proposed framework consists of two modules: an Information Collector and a Decision Maker. The Information Collector provides decision-makers with detailed information on classification results, including the features of the object and its shadow, as well as the classifier’s confidence in each classification. The Decision Maker uses this information to make more accurate and reliable decisions by considering both the features of the object and the potential influence of background information.
The authors demonstrate the effectiveness of their framework through experiments using a multilayer case. They show that GNN-based ATR outperforms traditional XAI methods in terms of accuracy and explainability. Furthermore, they illustrate how the proposed framework can help decision-makers identify misclassifications and improve their decision-making process by considering both the object’s features and background information.
In summary, this article presents a novel approach to XAI in multilayer cases that combines the strengths of GNNs with the explainability of XAI. The proposed framework provides decision-makers with more accurate and reliable information to support their decision-making processes by considering both the features of the object and background information.
Computer Science, Computer Vision and Pattern Recognition
Unveiling Decision-Making Secrets: A GNN-Based Approach to Explainable ATR
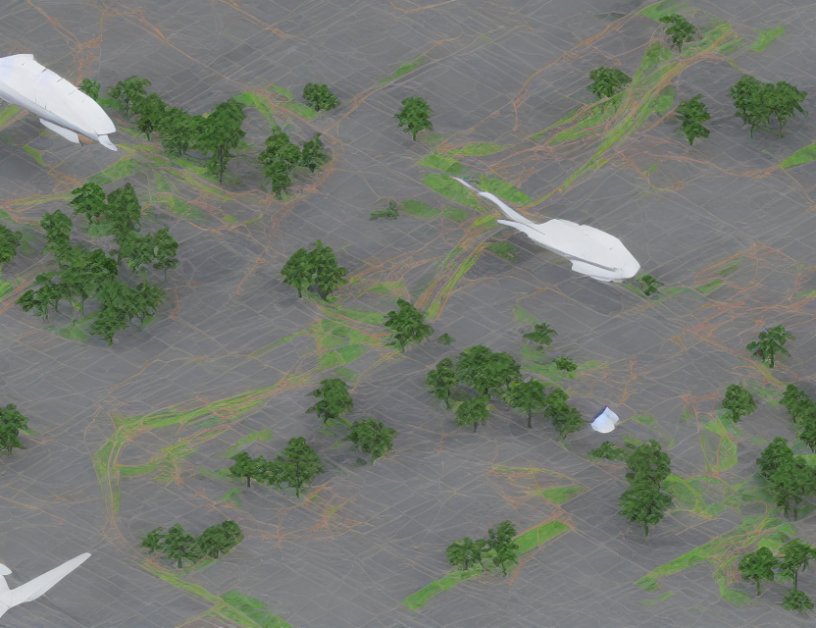